Our mission is to determine why people fall (and why they don’t) with the goal of remediating balance impairment. We pursue this objective by developing novel tools to assess balance proficiency and fall risk, identifying neuromechanical mechanisms of “better” balance, and optimizing rehabilitation interventions that improve walking balance. Our lab is based in the Department of Kinesiology and Nutrition in the UIC College of Applied Health Sciences.
Research approach
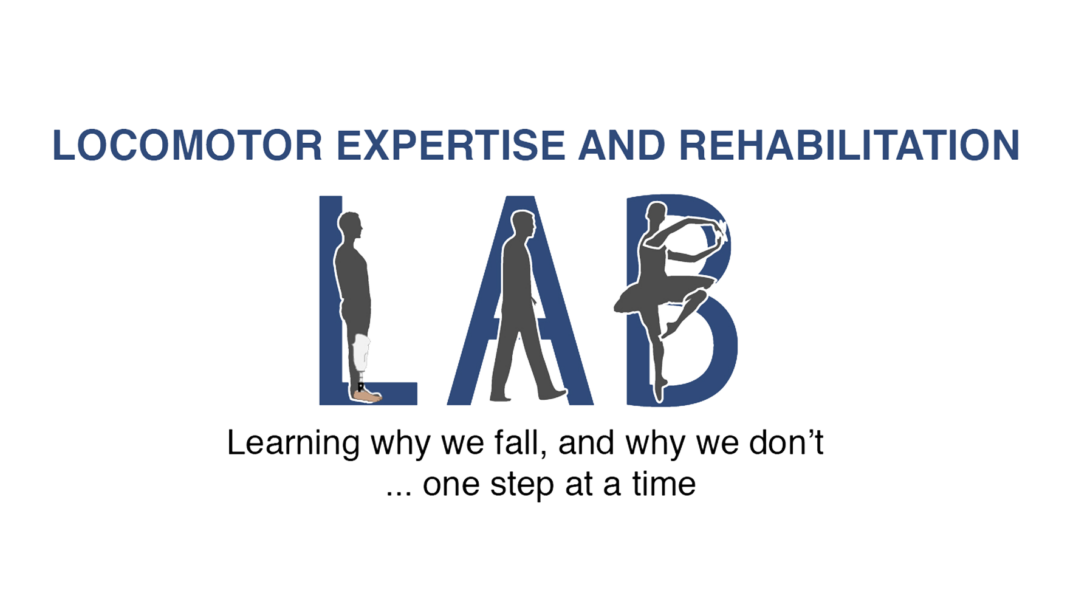
Over 50% of people with lower limb amputation (LLA) report one or more falls a year, placing them at risk for injury, reduced mobility, and diminished quality of life. With diabetes expected to double the number of amputations to 3.6 million by the year 2050, and the prevalence of falls remaining unchanged in people with LLA over the past 20 years despite advances in prosthetic technology, new approaches to address this serious health problem are required. In service of our long-term goal, to reduce preventable and consequential falls in people with LLA, our research integrates three key areas:
- epidemiology of falls
- clinical balance assessment
- biology of limb loss
Our efforts in each of these area to date include:
- the development of a structured, meaningful, amputee-specific fall survey to facilitate accurate reporting and enhance the characterization of fall frequency, circumstances, and consequences,
- the development, validation, and application of simple clinical tests to improve fall risk prediction, and
- the identification of modifiable deficits in muscle structure and function to provide surgeons, engineers, and therapists with biological targets their procedures, devices, and interventions can address.